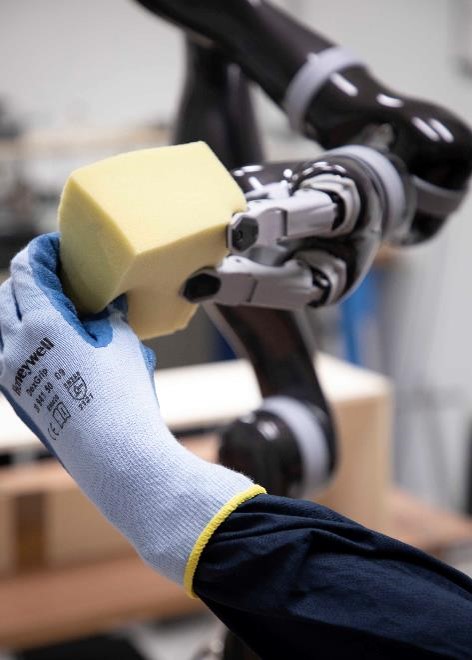
Objectives
The APRIL project aims to create autonomous, dexterous and market-oriented robot prototypes that will provide new ways of automating the manufacturing and processing of flexible and deformable materials.
This will enable new ways of automation (semi- or fully-automated tasks) in manufacturing lines that produce, assemble or handle different types of flexible or deformable materials—from pillows to delicate food products.
Our robots will use multi-sensor feedback to interact with their environment and human workers (see below). This includes tactile and visual feedback and a complex grasp-planning controller, allowing the robots to execute intricate, dexterous grasping and manipulation tasks with various objects made of different materials.
The APRIL approach allows to continuously transfer the learning of skills and abilities between areas of application (e.g., food, appliances, or passports manufacturing) by connecting the robots to a cloud-based knowledge database (see below). This database comprises knowledge related to safety and general object manipulation as well as domain-specific, dedicated knowledge. Connection to this database will allow the APRIL robots to be easily reprogrammed and repurposed for different tasks, thus becoming a cost-effective solution.
The robots will be uncaged and will be able to recognise gestures, posture, and facial expressions, and they will attempt to predict future actions of the human worker, to guarantee safety and ergonomics during human-robot collaborative tasks (see below).
APRIL prototypes will be validated in six different real-world scenarios, the APRIL use cases. These will explore potential business models for its robot prototypes, including leasing schemes.
To see APRIL results, have a look at our Research Papers, Patents (available soon), and Public Deliverables (available soon).
SPECIFIC OBJECTIVES
… with high dexterity for manipulation of flexible materials by Y3 of the project. This prototype will be scalable in functions and connected as a plug-in to an existing knowledge base in the cloud, achieving high-level reasoning capabilities.
APRIL robots stand out due to their high dexterity and sophisticated manipulation abilities for changing position and orientation of objects. The robots autonomously decide how to interact with different soft and flexible materials and how to respond to environmental changes and unforeseen events. This allows the robots to be easily adapted to changing tasks and environments.
… by acquiring different skills to manipulate at least three types of flexible materials (food, plastics, paper) and five different characteristics (texture, size, shape, weight, colour, material composition) of the flexible materials.
G&M in industrial contexts are easily performed by human workers but often challenging for machines. Therefore, human workers perform tasks that require high dexterity and manipulation skills in factories. However, repetitive tasks lead to fatigue, boredom, excessive strain on the body, etc., reducing performance. APRIL researches and develops better G&M abilities for different soft objects in industrial processes, alleviating the burden on human workers and ensuring a constant high quality of production.
… to enable grasp planning in complex scenes.
An intelligent high-level controller will be developed, allowing the robot to act autonomously in complex and changing production environments and collaborate with human workers. The KREM will improve the robot’s capabilities to continually adapt actions and plans to the current situation through its link with spatial reasoning, scheduling of consumable resources, reasoning about the human worker’s future course of actions, or coordinating multiple robots.
… for recognition and modelling of objects and environment.
A Perceptual Engine Module (PEM) uses optical sensors and semantic-enabled data processing for the recognition and modelling of the surrounding environment. A particular challenge that APRIL aims to solve is the characterisation of transparent objects. Since most visual sensors have great difficulty with this task, a new set of algorithms is being developed to obtain the information required to grasp and manipulate these objects. Successful recognition and manipulation of such objects is used to improve these capabilities in the future (using faster R-CNN along with decision trees).
… between humans and robots.
Safety and ergonomics of human workers during the production process are built in. Proactive measures and countermeasures are based on a neuromorphic architecture, including cameras for object and context recognition, as well as vision-based gesture recognition (pose and facial expression).
… through six use cases in five countries.
To ensure its real-world relevance and capabilities, the APRIL prototype will be tested and validated in six different manufacturing domains—food, home appliances, textile, electronics, shoes, as well as quality control during passport production—in five countries: Spain, Portugal, Italy, Hungary and Turkey.
… for a sustainable adoption of APRIL outputs.
This includes evaluating the provision of APRIL robots as a service (RaaS) for handling soft and flexible materials. APRIL aims to build a competitive advantage through the Federated Knowledge Base. With this, the APRIL robot can be used in a “plug-and-play” fashion and rapidly adapt to new tasks and objects thanks to cloud-based optimisation.